6 semanas
2–4 horas por semana
A tu ritmo
Avanza a tu ritmo
Gratis
Verificación opcional disponible
Hay una sesión disponible:
¡Ya se inscribieron 52,311! Una vez finalizada la sesión del curso, será archivadoAbre en una pestaña nueva.
Comienza el 20 nov
Termina el 30 nov
Deep Learning with Python and PyTorch.
Premios
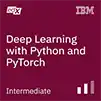
De un vistazo
- Institution IBM
- Subject Análisis de datos
- Level Intermediate
- Prerequisites
- Python & Jupyter notebooks
- Machine Learning concepts
- Deep Learning concepts
- https://www.edx.org/course/pytorch-basics-for-machine-learning
- Associated programs
- Professional Certificate en Deep Learning
- Language English
- Video Transcript English
- Associated skillsArtificial Neural Networks, Transfer Learning, Python (Programming Language), Deep Learning, Autoencoders, Machine Learning, Dimensionality Reduction, Convolutional Neural Networks, Principal Component Analysis, Feed Forward, PyTorch (Machine Learning Library)
¿Quién puede hacer este curso?
Lamentablemente, las personas residentes en uno o más de los siguientes países o regiones no podrán registrarse para este curso: Irán, Cuba y la región de Crimea en Ucrania. Si bien edX consiguió licencias de la Oficina de Control de Activos Extranjeros de los EE. UU. (U.S. Office of Foreign Assets Control, OFAC) para ofrecer nuestros cursos a personas en estos países y regiones, las licencias que hemos recibido no son lo suficientemente amplias como para permitirnos dictar este curso en todas las ubicaciones. edX lamenta profundamente que las sanciones estadounidenses impidan que ofrezcamos todos nuestros cursos a cualquier persona, sin importar dónde viva.Este curso es parte del programa Deep Learning Professional Certificate
Más informaciónInstrucción por expertos
6 cursos de capacitación
A tu ritmo
Avanza a tu ritmo
7 meses
2 - 4 horas semanales
¿Te interesa este curso para tu negocio o equipo?
Capacita a tus empleados en los temas más solicitados con edX para Negocios.