Lo que aprenderás
- Bayes’ Theorem. Differences between classical (frequentist) and Bayesian inference.
- Posterior inference: summarizing posterior distributions, credible intervals, posterior probabilities, posterior predictive distributions and data visualization.
- Gamma-poisson, beta-binomial and normal conjugate models for data analysis.
- Bayesian regression analysis and analysis of variance (ANOVA).
- Use of simulations for posterior inference. Simple applications of Markov chain-Monte Carlo (MCMC) methods and their implementation in R.
- Bayesian cluster analysis.
- Model diagnostics and comparison.
- Make sure to answer the actual research question rather than “apply methods to the data”
- Using latent (unobserved) variables and dealing with missing data.
- Multivariate analysis within the context of mixed effects linear regression models. Structure, assumptions, diagnostics and interpretation. Posterior inference and model selection.
- Why Monte Carlo integration works and how to implement your own MCMC Metropolis-Hastings algorithm in R.
- Bayesian model averaging in the context of change-point problem. Pinpointing the time of change and obtaining uncertainty estimates for it.
Información general del programa
Capacitación de la mano de expertos
2 cursos de capacitación
A tu ritmo
Avanza a tu ritmo
3 meses
5 - 10 horas por semana
338 US$
Para obtener la experiencia completa del programa
Cursos en este programa
Certificación Profesional en Bayesian Statistics Using R de UCx
- Introduction to Bayesian Statistics Using R
- Advanced Bayesian Statistics Using R
- Perspectivas de empleo
Conoce a tu instructor de University of Canterbury (UCx)
Expertos de UCx comprometidos con el aprendizaje en línea
Comienza con data science
Explorar otros cursos de data science¿Quieres avanzar en tu carrera profesional, obtener un título o aprender algo por motivos personales? edX tiene los cursos que necesitas
Inspiradores
Impulsa tu carrera profesional con programas de crédito respaldados por universidades y certificados verificados.Prácticos
Estudia y demuestra tu conocimiento a tu ritmoFlexibles
Prueba un curso antes de pagarAlentadores
Estudia con compañeros universitarios y colegas de todo el mundo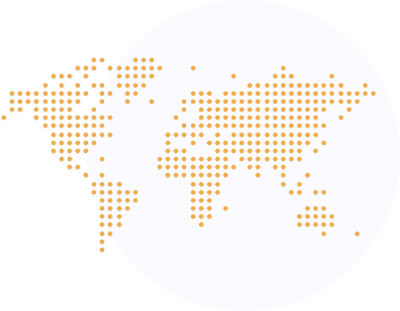